[ad_1]
The team unveiled two of these innovative chips at the IEEE International Solid-State Circuits Conference (ISSCC) 2024, the Olympics of the integrated circuit (IC) industry.
ISSCC is an annual global gathering on solid-state circuits that brings together the best researchers, engineers, and experts to discuss new developments and the future of chip technology. This year’s conference was held in San Francisco from February 18th to 22nd.
China’s AI gap with the US is widening: ‘We’re all very concerned’
China’s AI gap with the US is widening: ‘We’re all very concerned’
Traditional chips also have high energy consumption during wake-up and tend to have frequent false wake-ups, making them less energy efficient.
Zhou’s team proposed a new architecture that overcomes these limitations through multiple optimizations, including a dynamic calculation engine, an adaptive noise suppression circuit, and an integrated keyword and speaker recognition circuit.
“This chip achieves recognition energy consumption of less than 2 microjoules per instance and achieves accuracy of 95 percent in quiet scenes and more than 90 percent in noisy environments, setting new global benchmarks for both energy efficiency and accuracy. The UESTC website states:
In a demonstration of the system, the 1 square centimeter (0.155 square inch) chip was embedded in a 3cm x 3cm microcontroller unit inside a toy car to control its movements.
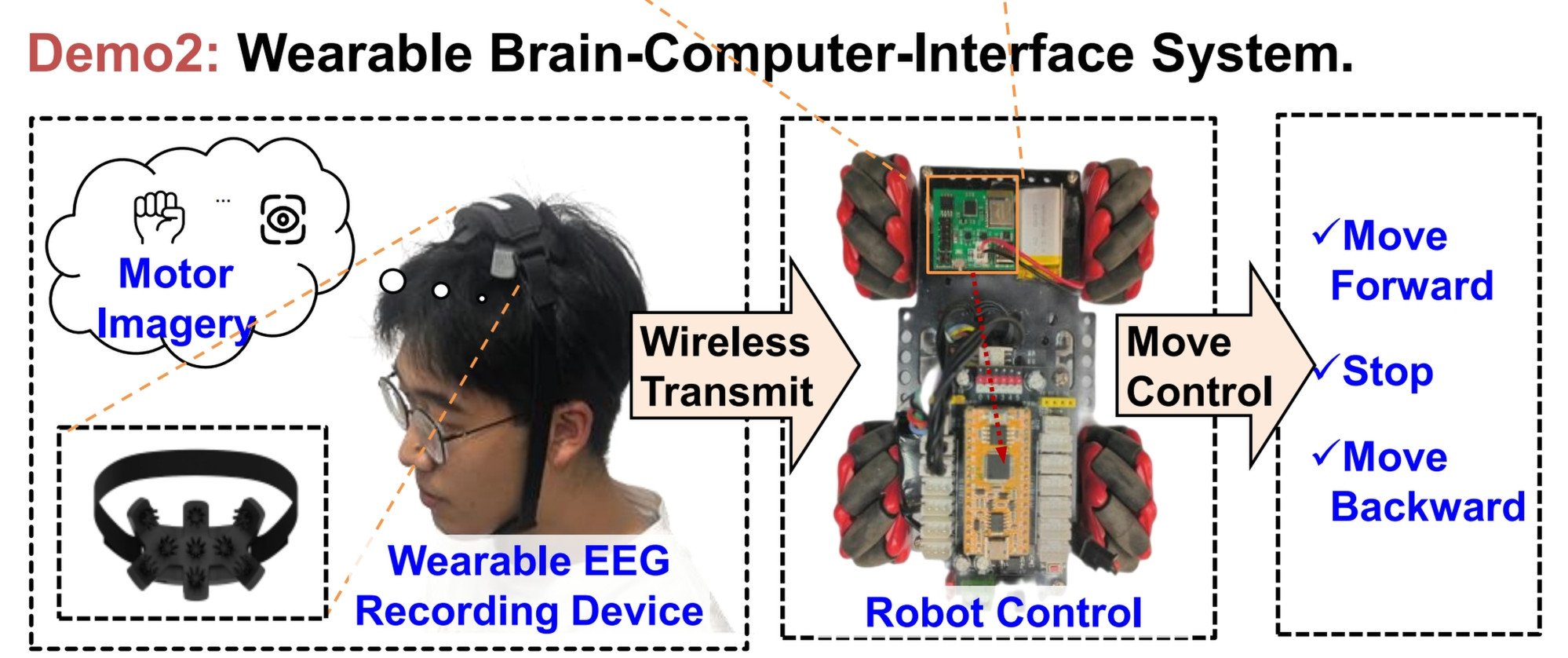
“Existing designs rely on extensive patient seizure data for training to achieve high accuracy, but this process is time-consuming and costly due to the low incidence of seizures and the need for hospitalization. ,” the report states.
To solve this particular challenge, researchers optimized a zero-shot retraining algorithm so that the pre-trained AI model can accurately predict invisible data without collecting patient seizure signals. and achieved an accuracy of over 98%.
Before use, the patient simply wears the device in its natural state and calibrates it for 2 minutes, allowing the device to recognize individual signal characteristics.
Further improvements in the feature extraction engine and on-chip learning engine result in the chip’s average recognition energy consumption of only approximately 0.07 microjoules, making it the most energy-efficient design of its kind internationally.
The official report said it improved accuracy by 10 percent and reduced energy consumption by more than 90 percent compared to another chip presented at last year’s conference.
In the demonstration at ISSCC, real-time user EEG signals collected from a wearable brain-computer interface device were transmitted to a test board via Bluetooth. The chip has been reconfigured to identify imaginary motor commands, allowing it to control movements such as moving the robot forward, stopping, and retreating.
[ad_2]
Source link